Daniel Langkilde, CEO and Co-founder of Kognic - AITech Interview
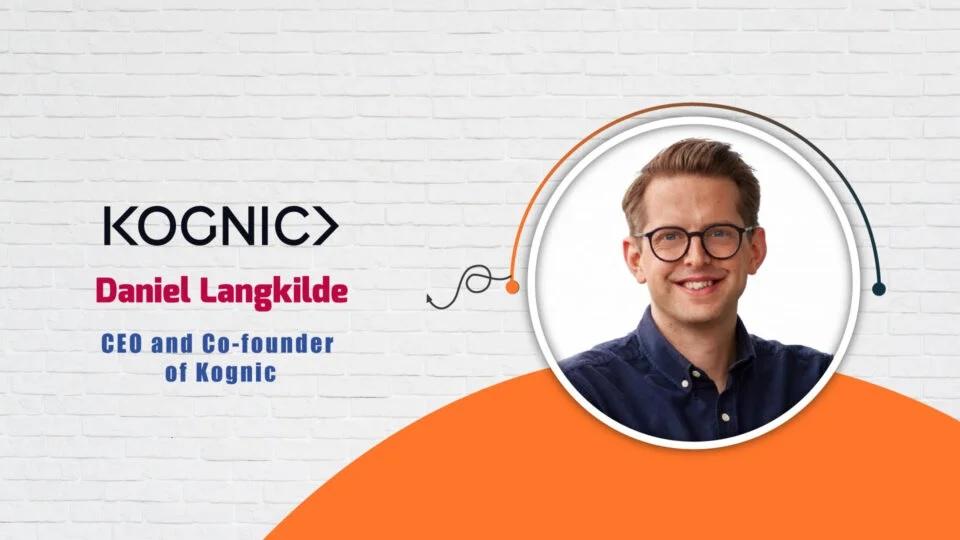
To start, Daniel, could you please provide a brief introduction to yourself and your work at Kognic?
I'm an experienced machine-learning expert and passionate about making AI useful for safety critical applications. As CEO and Co-Founder of Kognic, I lead a team of data scientists, developers and industry experts. The Kognic Platform empowers industries from autonomous vehicles to robotics – Embodied AI as it is called – to accelerate their AI product development and ensure AI systems are trusted and safe.
Prior to founding Kognic, I worked as a Team Lead for Collection & Analysis at Recorded Future, gaining extensive experience in delivering machine learning solutions at a global scale and I'm also a visiting scholar at both MIT and UC Berkeley.
Could you share any real-world examples or scenarios where AI alignment played a critical role in decision-making or Embodied AI system behavior?
One great example within the automotive industry and the development of autonomous vehicles, starts with a simple question: 'what is a road?'
The answer can actually vary significantly, depending on where you are in the world, the topography of the area you are in and what kind of driving habits you lean towards. For these factors and much more, aligning and agreeing on what is a road is far easier said than done.
So then, how can an AI product or autonomous vehicle make not only the correct decision but one that aligns with human expectations? To solve this, our platform allows for human feedback to be efficiently captured and used to train the dataset used by the AI model.
Doing so is no easy task, there's huge amounts of complex data an autonomous vehicle is dealing with, from multi-sensor inputs from a camera, LiDAR, and radar data in large-scale sequences, highlighting not only the importance of alignment but the challenge it poses when dealing with data.
Teaching machines to align with human values and intentions is known to be a complex task. What are some of the key techniques or methodologies you employ at Kognic to tackle this challenge?
Two key areas of focus for us are machine accelerated human feedback and the refinement and fine-tuning of data sets.
First, without human feedback we cannot align AI systems, our dataset management platform and its core annotation engine make it easy and fast for users to express opinions about this data while also enabling easy definition of expectations.
The second key challenge is making sense of the vast swathes of data we require to train AI systems. Our dataset refinement tools help AI product teams to surface both frequent and rare things in their datasets. The best way to make rapid progress in steering an AI product is to focus on that which impacts model performance. In fact, most teams find tons of frames in their dataset that they hadn't expected with objects they don't need to worry about – blurry images at distances that do not impact the model. Fine-tuning is essential to gaining leverage on model performance.
To Know More, Read Full Article @ https://ai-techpark.com/aitech-interview-with-daniel-langkilde/
Read Related Articles:
- Авто, мото
- Кейтеринг
- Досуг, развлечения
- Животные
- Красота, здоровье
- Образование, репетиторы
- Спорт и тренеры
- Строительство и ремонт
- Товары и магазины
- Туризм и отдых
- Финансы и страхование
- Литература
- Музыка
- История
- Политика
- Религия
- Искусство
- Кино
- Театр
- Хорошее здоровье
- Аксессуары
- Бизнес
- Разное