Mitigating Algorithmic Bias in AIOps: Strategies for Fairness and Transparency
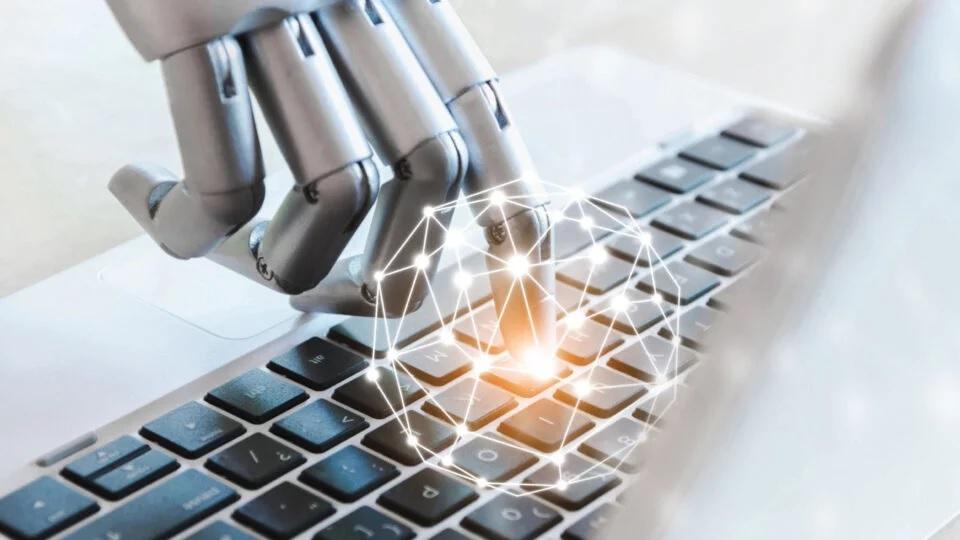
The business world is increasingly turning to artificial intelligence (AI) systems and machine learning (ML) algorithms to automate complex and simple decision-making processes. Thus, to break through the paradigm in the field of IT operations, IT professionals and top managers started opting for AIOps platforms, tools, and software, as they promised to streamline, optimize, and automate numerous tasks quickly and efficiently. However, there are a few shortcomings, like algorithmic bias, that have been a major concern for IT professionals and other employees in the company.
Key Technologies in Addressing Algorithmic Biases
With the use of cutting-edge AIOps technologies, IT professionals can understand and explore the algorithmic biases in the system. Thus, here are a few key technologies that will help you detect such issues:
Time Series Analysis
When having abundant data, time series analysis emerges as a crucial tool in AIOps as it records data over time by tracking users' behavior, network activity, and system performance. Algorithms should represent temporal dependencies, trends, and seasonality to detect biases effectively. AIOps uses a time series analysis method that includes autoregressive models, moving averages, and recurrent neural networks to examine the time-stamped data for deviation and identify abnormalities quickly.
Unsupervised Learning Techniques
Unsurprised learning is an essential component of AIOps for detecting algorithm biases and unwanted labeled data, which is necessary for traditional supervised learning but with limited knowledge. To discover issues, techniques like clustering and dimensionality reduction are crucial in revealing hidden structures within data.
Machine Learning and Deep Learning
The use of ML and deep learning techniques helps in regulating the different established standards, which enables the AIOps system to learn patterns and relationships from complicated and massive data and also enables it to detect analogous biases.
While not all scenarios involving algorithmic bias are concerning, they can have major negative effects when the stakes are high. We have seen that algorithmic prejudice poses a severe threat to human privacy, with lives, livelihoods, and reputations at stake, as well as concerns about data integrity, consent, and security. Integrated AIOps ensure that IT professionals and managers avoid bias and unfairness in their AI and ML models by considering any subjective elements associated with people, locations, products, etc. in their training data and models.
To Know More, Read Full Article @ https://ai-techpark.com/algorithmic-biases-solutions/
Read Related Articles:
Ethics in the Era of Generative AI
Generative AI for SMBs and SMEs
Maximize your growth potential with the seasoned experts at SalesmarkGlobal , shaping demand performance with strategic wisdom.
- Auto, moto
- Catering
- Leisure, entertainment
- Animals
- Beauty, health
- Education, tutors
- Sports and coaches
- Construction and repair
- Products and stores
- Tourism and recreation
- Finance and Insurance
- Literature
- Music
- History
- Politics
- Religion
- Art
- Movie
- Theater
- Wellness
- Accessories
- Business
- Various